-
Product Management
Software Testing
Technology Consulting
-
Multi-Vendor Marketplace
Online StoreCreate an online store with unique design and features at minimal cost using our MarketAge solutionCustom MarketplaceGet a unique, scalable, and cost-effective online marketplace with minimum time to marketTelemedicine SoftwareGet a cost-efficient, HIPAA-compliant telemedicine solution tailored to your facility's requirementsChat AppGet a customizable chat solution to connect users across multiple apps and platformsCustom Booking SystemImprove your business operations and expand to new markets with our appointment booking solutionVideo ConferencingAdjust our video conferencing solution for your business needsFor EnterpriseScale, automate, and improve business processes in your enterprise with our custom software solutionsFor StartupsTurn your startup ideas into viable, value-driven, and commercially successful software solutions -
-
- Case Studies
- Blog
7 Web Development Trends That Will Shape the Industry
This year is going to be a year of better cybersecurity, great potential for SEO ranking, and improved user experiences. If you’re looking for web development solutions to improve your product’s usability, performance, and ranking, check out our list of the ten most prominent web development trends. We’ve teamed up with RubyGarage experts from different fields to get their opinions about trends in web development and share them with you. Enjoy!
#1 Accelerated Mobile Pages (AMP)
Accelerated Mobile Pages (AMP) are already here. It’s now two years since the public launch of Google’s AMP project, and it’s gaining momentum. AMP is an open-source initiative that enables pages to load super-fast. AMP strips web pages down to the basics, removing scripts that slow them down. In a nutshell, AMP improves the overall user experience and website visibility in search results.
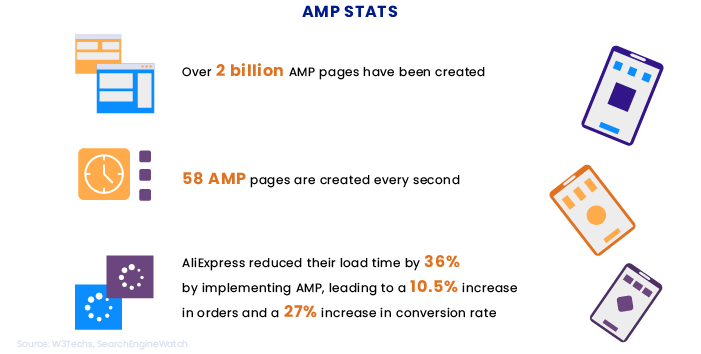
An AMP consists of the three following elements:
- AMP HTML (standard HTML with AMP-specific extensions)
- AMP JS (fast-loading JavaScript that loads the repository)
- AMP CND (an optimized network created to cache pages and quickly adapt them to AMP code)
The increasing popularity of AMP may be attributed to the fact that Google’s mobile-first index outweighs Google’s traditional desktop index. According to Statcounter, almost 59% percent of all internet traffic comes from mobile phones and tablets. So as search engine optimization (SEO) moves toward mobile devices, the adoption of AMP will accelerate. Let’s consider the advantages of moving to AMP for a website:
Faster load speed
A usual web page takes about three seconds to load, while an AMP page can load in just half a second. Faster loading improves the user experience as a whole. Websites that have moved to AMP show amazing results in reduced bounce rates and increased conversions. For example, Walmart has reported that AMP reduced their mobile load time by one second and resulted in a 2 percent increase in conversions. Yahoo’s AMP saw a 40 percent load time decrease and, as a result, a 9 percent increase in traffic.
Improved mobile ranking
Although AMP support isn’t an official ranking factor, it can positively influence mobile rankings thanks to faster load speed and greater convenience. With time, AMP web pages will overtake traditional pages in search results.
Better server performance
AMP is a great solution for websites that generate tons of mobile traffic. Switching to AMP reduces the load on a website server and improves performance.
To understand the difference between traditional web pages and AMP pages, let’s look at the following example:
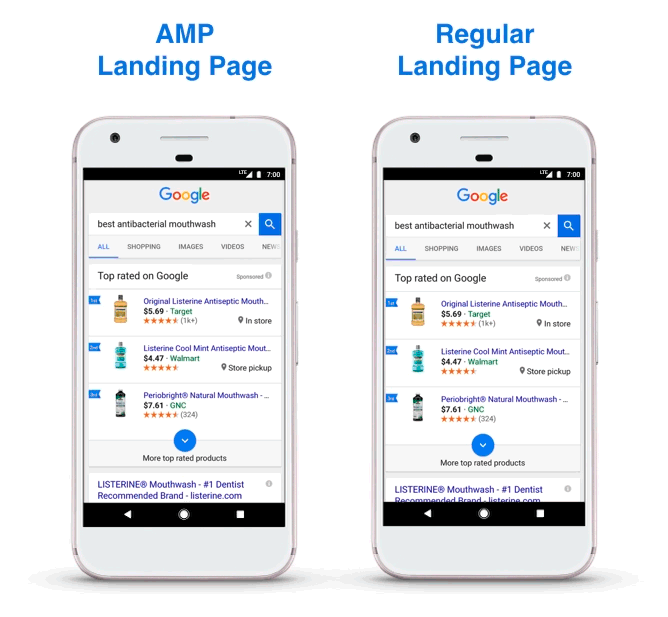
AMP helps websites rank higher in SERP results, increase conversion rates, and load pages in less than a second. Some large websites like The New York Times, The Wall Street Journal, BMW, Yahoo, and Reddit have already successfully adopted this technology.
#2 Progressive web apps (PWAs)
As almost 60% of internet traffic comes from smartphones and tablets at this point, there’s a well-established need for businesses to target customers on mobile devices. Businesses that want to attract mobile-based users have three ways to do so: build a native app, create a responsive website, or develop a progressive web application (PWA).
Native apps feature a perfect user experience but at the same time have higher adoption barriers. Users are required to download the app to start using it. Mobile websites are less efficient from the user experience perspective but they’re quicker to access. Bridging the gap between these two options would be the most beneficial solution for businesses. And that’s where progressive web apps come into play. A progressive web app (PWA) functions just like a native app but doesn’t oblige users to download it from the app store. A PWA is a mobile application delivered through the web that’s always accessible and loads instantly. This technology consists of three main components:
- Application shell architecture (for quick loading with server workers)
- Server workers (for offline support and background tasks)
- Web app manifest (for native-like features)
Let’s consider the advantages of PWAs in detail:
- PWAs are efficient, as they work on-demand and don’t take up much storage space.
- PWAs can work offline.
- PWAs match the mobile-first approach; they function on mobile devices without any issues.
- With a PWA, a business doesn’t need to create a separate native app, saving up to 75 percent on development and maintenance costs.
- A PWA loads two to three times faster than a usual app and, as a result, reduces server load.
The idea of PWAs sounds impressive, but are there any success stories of applying this technology? Sure! Look at the following stats of giant companies who have already launched PWAs.
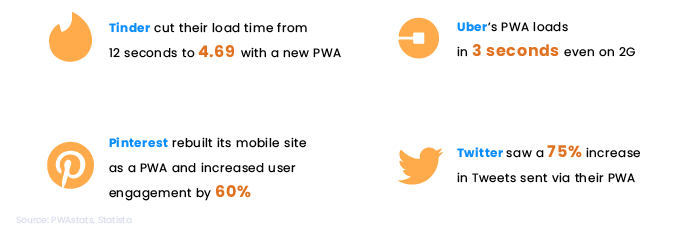
Native apps and mobile websites have their pluses and minuses, but PWAs have already proved the best alternative. The reason I’m so impressed by this technology is that it’s capable of providing similar functionality across all devices.
This technology will continue to gain traction in the future as statistics show a considerable increase in user experience and engagement when using PWAs.
#3 Voice search optimization
Voice search technology is growing at a tremendous rate. Traditional search remains strong, but it’s reported that more and more people are choosing to speak to assistants rather than type.
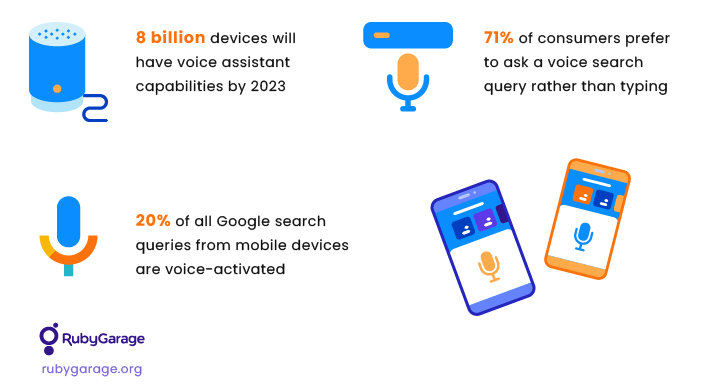
Voice search technology allows users to search by asking a question to a smart device. Today, this technology is affecting a lot of spheres, especially ecommerce. Voice commerce lets users look for products and complete purchases much faster and more efficiently than manual search. Amazon’s Alexa, Google Assistant, Apple’s Siri, and Microsoft’s Cortana are the most popular voice search assistants at this point.
You probably wonder how this technology can impact your business and what advantages you might get from optimizing your website for voice search. Here are the answers.
- Higher ranking. Search engines like Google emphasize websites that are optimized for voice search, which means this technology can improve your SEO ranking.
- Better user acquisition. As voice search-optimized websites rank higher in SERP results, these websites have more chances to attract and engage customers.
- Increased traffic. Voice search optimization boosts your website traffic by making your website more visible and credible.
- Better user experience. Websites optimized for voice search provide faster results to a user’s search queries. Increased search speed results in better search productivity, a more pleasing user experience, and better user engagement.
Voice search technology is already in active use and has great prospects. The rise of mobile search and digital assistants make voice search optimization a must for websites in 2022 and beyond.
Voice search is an evolving trend that can’t be ignored by businesses if they want to have a competitive advantage and a higher SEO ranking in Google or any other search engine.
#4 Cybersecurity
The Cybersecurity Ventures report claims that cybercrime will cost the world $10.5 trillion per year by 2025, up from $3 trillion in 2015. Cybersecurity has been a leading web development trend for the last few years.
Zero Trust security model
Zero Trust is a security concept that focuses on verifying everyone and everything trying to connect to a system before granting access. This strategy boils down to never trust anyone when it comes to cybersecurity.
The Zero Trust security model works by continuously analyzing an individual’s behavior to prevent potential threats. The Zero Trust system calculates risk factors and then generates a risk score. And if this risk score surpasses a threshold, the user will be either disconnected or obliged to pass multi-factor authentication.
Gartner reports that companies worldwide spent about $150.4 billion on cybersecurity in 2021. Companies realize that existing technologies aren’t secure enough and find the Zero Trust model one of the most effective.
GDPR
The General Data Protection Regulation (GDPR) is a data protection reform that went into effect in May 2018 in the European Union. This EU regulation was created to regain control of data security and manage data protection. Forrester research states that companies that have already embraced GDPR report improvements in customer trust and experience overall business growth.
Data protection requirements are growing among European countries and beyond. The California Consumer Privacy Act (CCPA) came into force in 2020. The GDPR has great potential in the cybersecurity field, as it has already proved its efficiency in Germany, the UK, and other European countries.
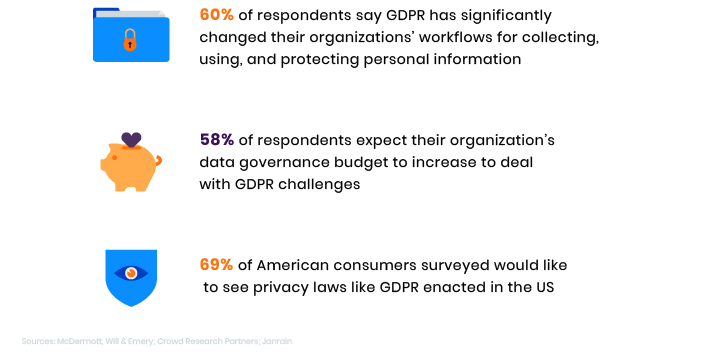
Biometric security
MarketsandMarkets projects that the biometric systems market will be worth about $82.9 billion by 2027, growing at a CAGR of 14.1%. Companies will use biometric tools to protect their data, as this approach significantly increases the effectiveness of cybersecurity. Some time ago, people didn’t feel comfortable sharing their biometric data through technologies such as retina and fingerprint scanning. But today, with the rise of mobile devices, consumers feel safe unlocking their smartphones with FaceID and fingerprint identification.
Biometrics has already become a standard feature for mobile devices. Biometric authentication provides increased convenience and stronger protection, as this technology proves a person’s identity with a higher level of certainty than password authentication.
AI chatbots
The ongoing COVID-19 pandemic has led companies to digitize their customer service to become more independent from human resources, cut operational costs, and make their services accessible to customers 24/7. According to Business Insider, nearly 40% of internet users globally prefer interacting with chatbots. On the other hand, using AI chatbots is also beneficial for businesses. Their adoption could save the banking, retail, and healthcare sectors up to $11 billion annually by 2023.
More and more big companies are using chatbots as one of their leading customer interaction channels, including Starbucks, eBay, LinkedIn, and British Airways. Key market players such as Google, Microsoft, and Facebook continue investing in AI-based chatbot technologies, making their solutions more functional and more human-like. Because of this, AI-based chatbots are likely to remain one of the biggest web development trends for the next few years.
#6 Serverless architecture
The web development industry is evolving to reduce cases of system overloading, prevent data loss, and cut development costs. Serverless technology meets all of these goals. Cloud technology allows companies to run any type of apps or backend services without needing to manage the infrastructure. There’s no need to upgrade servers to cope with growing data loads, as all such issues are managed by the cloud service provider.
The serverless architecture market is expected to reach $21.1 billion by 2025, up from $7.6 billion in 2020 and growing at a CAGR of 22.7%. This indicates growing demand for serverless technology and its wider adoption in the years to come.
#7 Web design with a business-driven approach
Web development and web design go hand in hand today. So we decided to share some design trends as well. According to a report by McKinsey, UX design is driving business success.
Now designers are creating more empathy for businesses – not just for users.
More than ever, design teams will strive to make their design approaches research-driven, measure the efficiency of design, and optimize design solutions to solve real business problems.
Among design trends connected to web development, voice UI is a talking point. With the impressive growth of smart speakers and voice search technology, the design industry will need to adopt user-friendly voice interfaces.
One more interesting trend to consider is device-agnostic design, a modern-day approach to responsive design. Device-agnostic design says to forget about devices and focus on user needs. In 2019, designers will think in terms of providing the best possible experience for users across all devices at any point in the user journey.
Summing up
On the whole, 2019 is going to be another exciting year for new technologies in the web development industry. If you want to set your business apart this year, you’d better consider some trends from the list above and find a useful technology to apply. Don’t hesitate to share your thoughts on this topic in the comments below!

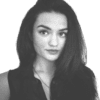

