-
Product Management
Software Testing
Technology Consulting
-
Multi-Vendor Marketplace
Online StoreCreate an online store with unique design and features at minimal cost using our MarketAge solutionCustom MarketplaceGet a unique, scalable, and cost-effective online marketplace with minimum time to marketTelemedicine SoftwareGet a cost-efficient, HIPAA-compliant telemedicine solution tailored to your facility's requirementsChat AppGet a customizable chat solution to connect users across multiple apps and platformsCustom Booking SystemImprove your business operations and expand to new markets with our appointment booking solutionVideo ConferencingAdjust our video conferencing solution for your business needsFor EnterpriseScale, automate, and improve business processes in your enterprise with our custom software solutionsFor StartupsTurn your startup ideas into viable, value-driven, and commercially successful software solutions -
-
- Case Studies
- Blog
7 Exciting Uses of Machine Learning in FinTech
A breakthrough in machine learning would be worth ten Microsofts.
Machine learning (ML) has moved from the periphery to the very center of the technology boom. But which industry is best positioned - with the huge data sets and resources - to take advantage of machine learning? According to research by PwC, this industry is finance.
Machine learning can significantly contribute to your FinTech project’s success by increasing data protection and customer engagement, among other things. In this article, we review the most prominent use cases of machine learning in FinTech and provide examples.
#1 Algorithmic trading
Algorithmic trading isn’t new, but it’s still a very effective strategy that many financial companies use to automate their financial decisions and increase trades. According to Techfunnel, 73 percent of daily trading worldwide is carried out by machines in 2017. Almost every major financial company invests in algorithmic trading as the frequency of trades executed by machine learning technology is impossible to replicate manually.
The following table shows the difference between manual and algorithmic trading:
Algorithmic trading | Manual trading |
---|---|
Free of emotions | Involves human emotions |
Rules are pre-defined | No predefined rules |
No need to monitor the market | Need to monitor the market all the time |
Slippage reduces considerably | May lead to heavy slippages |
Bank of America has launched BofAML Express, a high-frequency trading platform. The capabilities of the platform are expected to be used not only by algorithmic traders but also by less technology-savvy customers. The platform’s activity is estimated to account for 2 to 3 percent of average daily US stock trading.
#2 Machine learning goes beyond predictive analytics
Many financial organizations today have moved from using traditional predictive analysis to using machine learning algorithms to forecast financial trends. With the help of machine learning, financial specialists can identify market changes much earlier than with traditional methods.
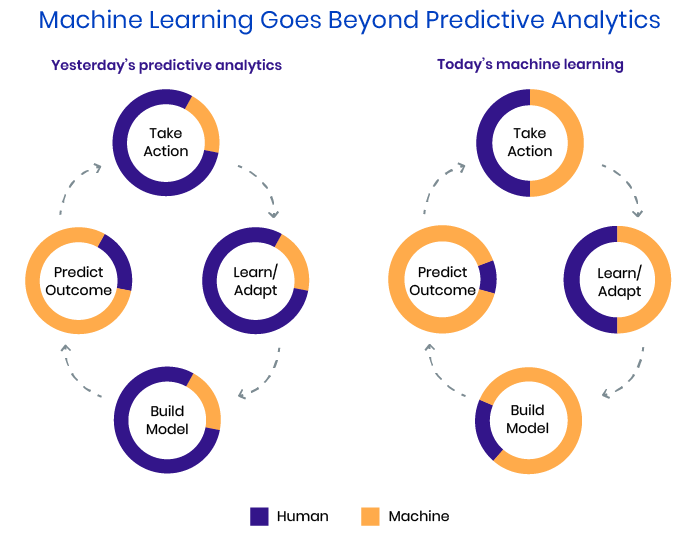
In the financial services industry, machine learning algorithms can predict market risk, reduce fraud, and identify future opportunities.
Machine learning technology is able to reduce financial risks in several ways:
- Machine learning algorithms are able to continuously analyze huge amounts of data (for example, on loan repayments, car accidents, or company stocks) and predict trends that can impact lending and insurance.
- Machine learning can also be applied to early warning systems. ML-enhanced early warning systems can be used by banks and other financial institutions to predict anomalies, reduce risk cases, monitor portfolios, and provide recommendations on what to do in cases of fraud.
#3 Fraud prevention and detection
With more technological innovations there are more risks of fraudulent transactions for financial organizations. The financial services industry is suffering from fraud-related losses more than any other industry. Almost 17 million organizations and customers in the US have experienced fraud according to Javelin’s 2018 Identity Fraud Report.
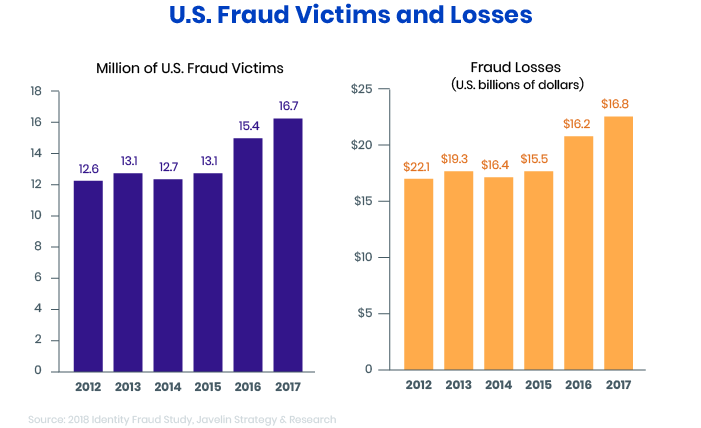
The financial industry takes two approaches to fraud detection and prevention: a rules-based approach (which requires manual work and human supervision) and a machine learning-based approach. In recent years, the financial services industry has been moving to ML-based approaches to detect fraudulent activity. Let’s look closer at the core features of these two approaches and clarify the benefits of machine learning.
Rules-based approach | ML-based approach |
---|---|
Long-term processing | Real-time processing |
Requires much manual work | Automatic detection of all possible anomalies |
Multiple verification steps that harm the user experience | Reduced number of verification measures |
Catches obvious fraudulent activities | Finds hidden fraudulent activities |
Below are some financial fields where machine learning is used for fraud detection.
Insurance
Fraudsters steal $80 billion a year across all branches of insurance according to the Coalition Against Insurance Fraud. Machine learning systems can detect unusual behavior, or anomalies, and flag them. Algorithms not only give detailed information on suspicious behavior but even suggest measures that can be taken to resolve situations and protect programs.
Banking
Electronic payments are extremely vulnerable to fraud. In banking, machine learning can delay potentially fraudulent transactions until a human makes a decision. Unlike humans, machines can weigh the details of a transaction and analyze huge amounts of data in seconds to identify unusual behavior.
Machine learning technologies are also used by banks for biometric user authentication. For example, ZOLOZ company has developed a technology using machine learning algorithms that makes it possible to use selfies to ensure the security of financial operations. The company’s Optical character recognition identifies a user by veins in the white of the eye and other unique eye features.
#4 Better targeting
According to recent research by L’atelier BNP Paribas, Millennials value transparency and convenience when dealing with financial services. They demand personalized services at their fingertips. The FinTech industry is trying to attract Millennials, a technology-savvy generation, with new technological trends. For instance, financial institutions are working on using machine learning technology and big data to replace human advisors with robotic advisors. Robo-advisors can not only attract Millennials but also eliminate a huge amount of processing costs for financial institutions.
For example, the Mylo FinTech app is using machine learning technologies to make it easier for Millenials to incorporate saving and investing into their daily habits. Upstart also considers Millennials an important market segment and uses machine learning to automate and facilitate borrowing.
#5 Better customer support
The times when bank clients stood in lines are over. With the help of modern technologies, banks and other financial institutions can make their services digital. Let’s have a closer look at examples of how machine learning can be applied to customer support:
Chatbots
Forrester research shows that 77 percent of bank clients in the United States consider saving customer time to be the most valuable aspect of good service.
By using technology like chatbots, machine learning helps financial institutions to solve customer issues immediately. For example, Bank of America introduced their Erica chatbot to provide customers with instant information about balances, transactions, and other related matters. Chatbots are beneficial in banking because they save money, increase customer engagement, and streamline customer support.
Personalized experience
Personalization is the key to building customer loyalty and trust toward any business or organization. Especially when dealing with finances, people value transparency and deep relationships with an institution they’ve chosen.
Machine learning algorithms can analyze customers’ data and predict what services they might like or give helpful advice. For example, Capital One has launched the Capital One Second Look program that can monitor expense patterns. After detailed analysis, this program can detect if a customer has been charged twice for the same product or service and notify them about it. Or it can analyze what tips the customer usually leaves at a restaurant and alert them if they’re overly generous. Programs like this make customers feel valued and motivate them to stay with a financial institution.
Sentiment analysis
Sentiment analysis, also called opinion mining, is a process of analyzing customers’ emotions, opinions, and attitudes toward other individuals, products, or services. In the financial industry, institutions use machine learning algorithms to analyze financial news from different sources and make predictions of possible stock market trends. The advantage of using technology for sentiment analysis lies in the ability to process huge amounts of data from different news channels in seconds.
So how exactly does this technology work? Sentiment analysis applications are programmed to classify all information as positive, negative, or neutral. For example, the words increase, growth, and successful can be defined as positive, while fall and risk are defined as negative. Based on this analysis, the technology makes predictions about financial trends.
#6 Help with underwriting services
Underwriting is the process of assessing risks that might be faced by an individual or company that wants to apply for life insurance or a loan, for example. But what if applicants purposely omit vital information about themselves or there’s no information about previous insurance deals? That’s where machine learning comes into play. Natural language processing (NLP) algorithms help financiers to better evaluate applicants by searching for personal information on social media, for example.
Machine learning algorithms can assess and predict the underlying insurance or loan trends that can influence the finance industry in future.
#7 Prediction of stock market changes
Everyone wants to trade smartly, especially in the stock market. The stock market is regarded as one of the best investment decisions in the twenty-first century. But how can you know which stocks are going to increase and which aren’t?
Machine learning technology analyzes past and real-time data about companies and predicts the future value of stocks based on this information. In addition, machine learning algorithms can even hunt for news from different sources to collect any data relevant to stock predictions.
The future of machine learning in the finance industry
It’s obvious that chatbots and robo-advisors are growing trends in the finance industry. But some financial institutions are predicting even more seamless communication with customers.
For example, Kasisto is already creating a chatbot that will be able to answer not only usual questions about balances and spending but also questions about customer’s past buying decisions and experiences.
It’s also possible that financial service providers will not only use chat functionality but also voice recognition. Customers will probably forget about irritating usernames and passwords to log in to their accounts as there will be facial and voice recognition or other methods of biometric authentication.
Wrapping up
Machine learning is playing an important role in the FinTech industry and is going to show even more potential in the future. Taking into account all use cases given above, it seems clear that machine learning algorithms are beneficial for financial institutions.
Feel free to start discussing FinTech trends in the comments below.